• DeepMind has developed Genie, a foundation model trained to produce 2D game levels based on either textual or image inputs.
• Genie simplifies the process of generating functional 2D environments for gaming purposes.
• The development of Genie opens up avenues for creating robots capable of accurately interpreting and navigating novel environments.
Google DeepMind’s Genie represents a breakthrough in generative modeling, seamlessly transforming basic image or text inputs into vibrant, interactive realms.
Trained on an expansive dataset exceeding 200,000 hours of in-game video footage, encompassing both 2D platformer gameplay and real-world robotics interactions, Genie has gained an unparalleled understanding of diverse environments and objects’ physics, dynamics, and visual aesthetics.
Documented in a comprehensive research paper, the refined Genie model boasts a staggering 11 billion parameters, empowering it to craft immersive virtual worlds from a variety of image formats or textual cues.
Genie’s versatility extends to transforming everyday scenes into playable 2D platform levels. Whether it’s your cozy living room or vibrant garden, simply provide Genie with an image, and it will seamlessly translate it into an engaging game environment.
Moreover, Genie’s capabilities go beyond images. You can even sketch a rudimentary 2D environment on a piece of paper and watch as Genie brings it to life, converting your doodles into fully playable game environments. With Genie, the boundaries between imagination and reality blur as creativity takes center stage.
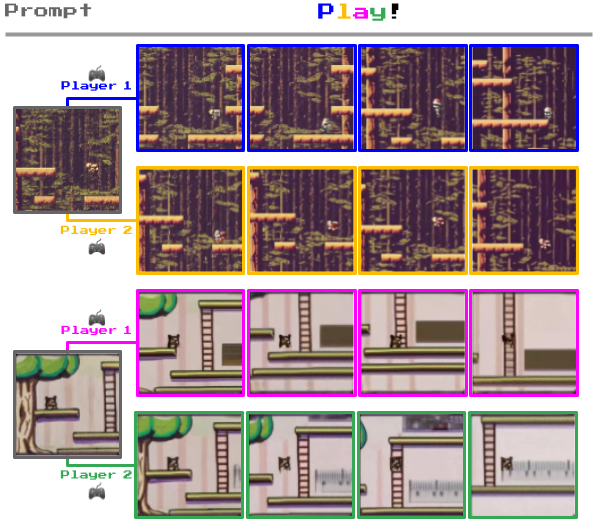
Genie stands out among world models for its unique feature allowing users to interact with generated environments frame by frame.
For instance, illustrated below is Genie’s capability to transform photographs of real-world settings into captivating 2D game levels.
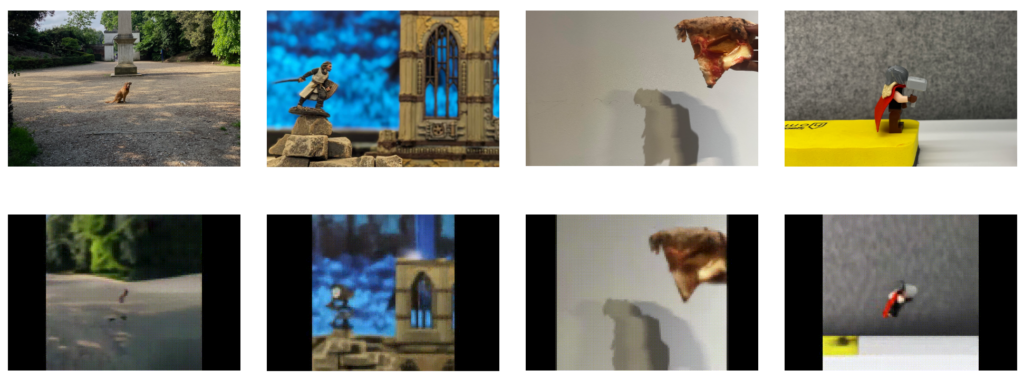
How Genie works
Genie, labeled as a ‘foundation world model,’ comprises three essential components: a spatiotemporal video tokenizer, an autoregressive dynamics model, and a straightforward, scalable latent action model (LAM).
Here’s a concise breakdown of Genie’s operation:
- Spatiotemporal Transformers: Genie employs specialized transformers to process video sequences, understanding visual data evolution over time, crucial for dynamic environment generation.
- Latent Action Model (LAM): Genie predicts actions within its generated worlds through a Latent Action Model, inferring potential actions between frames based on visual data, enabling control over interactive environments.
- Video Tokenizer and Dynamics Model: Genie uses a video tokenizer to condense video frames into manageable tokens. The dynamics model then predicts subsequent frame tokens, facilitating seamless generation of dynamic environments.
The DeepMind team elaborated on Genie’s potential, stating, “Genie could empower countless individuals to create personalized game-like adventures. This holds promise for fostering creativity, especially among children who can craft and explore their own imaginative realms.”
In a parallel experiment, Genie showcased remarkable proficiency in interpreting actions performed by real robot arms in videos. This remarkable ability hints at potential applications in robotics research, promising advancements in this field.
Tim Rocktäschel of the Genie team highlighted the limitless potential of Genie, stating, “It’s challenging to anticipate the various applications that may emerge. Our hope is that projects like Genie will ultimately equip individuals with new avenues for expressing their creativity.”
DeepMind acknowledged the cautious approach regarding the release of this foundational model, expressing in the paper, “We’ve made the decision not to share the trained model checkpoints, the model’s training dataset, or any examples from that dataset alongside this paper or the website.”
“We aim to foster further collaboration with the research (and gaming) community, ensuring that any future releases prioritize respect, safety, and responsibility.”
Utilizing Gaming for Simulating Real-World Scenarios
DeepMind has leveraged video games in numerous machine learning initiatives.
For instance, in 2021, DeepMind introduced XLand, a digital arena tailored for evaluating reinforcement learning (RL) strategies for versatile AI agents. Within XLand, AI models honed cooperative skills and problem-solving abilities by undertaking diverse challenges, such as manipulating obstacles within expansive game settings.
More recently, SIMA (Scalable, Instructable, Multiworld Agent) was developed to comprehend and enact human language instructions across varied gaming contexts and scenarios.
SIMA underwent training across nine video games with distinct skill requirements, ranging from fundamental navigation to advanced vehicle piloting.
Game environments serve as adaptable and scalable platforms for both training and evaluating AI models.
DeepMind’s involvement in gaming dates back to 2014-2015, highlighted by the development of algorithms capable of outperforming humans in games such as Pong and Space Invaders. Additionally, their groundbreaking achievement with AlphaGo, which triumphed over professional player Fan Hui on a standard 19×19 board, further underscores their expertise in gaming.