MIT and Google scientists introduced StableRep, a program that turns written captions into accurate images using pictures created by Stable Diffusion. This tool improves how neural networks generate images from text, with synthetic images aiding AI models in learning visual representations more accurately than real photos, according to the researchers.
MIT and Google scientists introduced StableRep, an AI model turning written captions into accurate images using Stable Diffusion. It enhances neural networks’ ability to generate images from text. The researchers believe synthetic images aid more accurate learning of visual representations compared to real photos. StableRep empowers researchers by training on diverse images, expanding the model’s understanding of visual prompts.
Researchers envision the emergence of an ecosystem of AI models, some of which will be trained on either real or synthetic data. Presently, efforts are focused on teaching the model to learn more about high-level concepts through contextual understanding and variability, instead of simply feeding it data.
StableRep: A Boost for AI Developers and Engines
Text-to-image models hinge on their ability to connect objects with words. When given a text prompt, these models must create an image that faithfully reflects the given description. To do so, they need to grasp the visual representations of real-world objects.
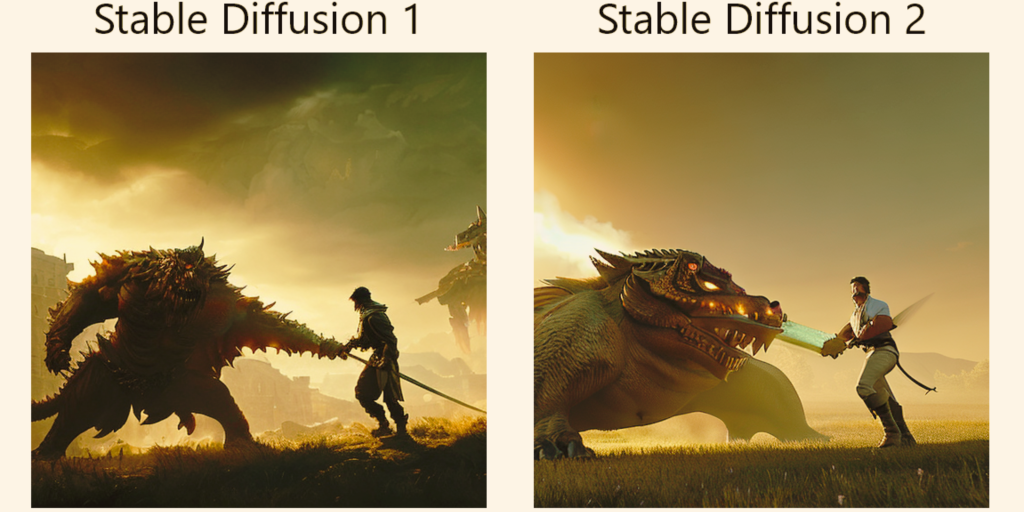
According to a recent pre-print paper on arXiv, StableRep outperforms SimCLR and CLIP in terms of learned representations using the same set of text prompts and corresponding real images on large-scale datasets, solely relying on synthetic images.
The paper continues, “When we further introduce language supervision, StableRep trained with 20 million synthetic images achieves better accuracy than CLIP trained with 50 million real images.”
SimCLR and CLIP are machine-learning algorithms employed for generating images from text prompts.
This new approach lets AI developers train neural networks with fewer fake images than real ones, and it produces better outcomes. Methods like StableRep hint at a future where text-to-image models could rely more on synthetic data for training, lessening the need for real images. This helps AI engines, especially when online resources are limited.